Titre : |
Filtermethoden zur fehlertoleranten kinematischen Positionsbestimmung |
Titre original : |
Filtering methods for error-tolerant kinematic positioning |
Type de document : |
Monographie |
Auteurs : |
J.G. Wang, Auteur |
Editeur : |
Munich : Universität der Bundeswehr |
Année de publication : |
1997 |
Collection : |
Schriftenreihe des Instituts für Geodäsie, ISSN 0173-1009 num. 52 |
Importance : |
135 p. |
Format : |
21 x 30 cm |
Note générale : |
Bibliographie |
Langues : |
Allemand (ger) |
Descripteur : |
[Vedettes matières IGN] Géodésie spatiale [Termes IGN] compensation [Termes IGN] erreur systématique [Termes IGN] filtre de Kalman [Termes IGN] GPS en mode cinématique [Termes IGN] positionnement cinématique [Termes IGN] variance
|
Index. décimale : |
30.61 Systèmes de Positionnement par Satellites du GNSS |
Résumé : |
(Auteur) The estimation process, the Kalman filter, is probably the most common optimal filtering technique for estimating the state of a linear system. In this thesis filtering methods for error-tolerant kinematic positioning are systemtic researched. This includes such topics as, statistical tests, the identification of gross errors in the observations, the identification of sensor systematic errors, the estimation of variance components and robust estimation in the Kalman filter.
With respect to theory, the analysis of reliability was incorporated into the theory of the Kalman filter as one of the most important parts on the quality control of a linear system. The method developed by Foerstner [19791 on the a-posteriori estimation of variance components was used for each type of observation and for all factors of the process noise in the Kalman filter. The difference between the FM-smoothing [Mayne, 1966; Fraser 1967] and the RTS-smoothing [Rauch, Tung, Striebel, 1965] was illuminated by objective functions and with the help of some examples. For the identification of gross errors a method was developed which makes use of additional parameters in the estimation process. The initialisation of these additional parameters can be introduced into the system as pseudo-observations, with expection zero and the very large variance. The robust method developed by Li [1983] was also realized in the Kalman filter.
With regard to the application of the Kalman filter to kinematic surveying, three models presented in this thesis were evaluated in the context of quality control with respect to accuracy, reliability, predictive residual errors, corrections of the observations and curvature of the filtered trajectories. Furthermore, statistical tests, methods for identification and compensation of gross errors in observations and methods for identification and compensation of sensor systematic errors and robust Kalman filter were investigated. All methods described in this thesis are put into practice with the help of simulated examples and were compared under the aspects mentioned above.
Chapter 1 gives an introduction to this thesis.
In Chapter 2 the fundamental principles of optimal linear filtering in discrete time is briefly introduced at first. Then the Kalman filter algorithm is derived using a least-squares approach. Afterwards it goes into the quality control in the Kalman filter. Especially the importance of the redundancies of the various kinds of observations is emphasized. The method of the a-posteriori estimation of variance components [Foerstner, 19791 will be used in the Kalman filter in this Chapter.
Chapter 3 presents the necessary fundamentals of kinematics
Chapter 4 is devoted to the establishment and evaluation of system models for kinematic positioning. The main aspects of Chapter 2 are realized for the filtering of land vehicle trajectories and are discussed in detail by three simulated examples. This performance analysis is very important for the practical application of the Kalman filter on kinematic positioning of land vehicle trajectories.
The next three Chapters, Chapter 5, 6 and 7, form two important parts of this thesis. The first one deals with the identification and compensation of gross errors in observations in Chapters 5 and 7. The second one deals with the identification and compensation of sensor systematic errors.
On one hand, the statistical tests and the recognizability of gross errors in observations are put up for the Kalman filter in Chapter 5. On the other hand, three proceedings are described for the identification and compensation of gross errors in the observations.
Chapter 6 concerns itself with the identification and compensation of sensor systematic errors. The considered systematic errors are constant, constant with a leap and time varying sensor systematic errors. The recognizability of the constant systematic error is also investigated in this Chapter.
Chapter 7 goes back to the handling of gross observation errors but only relating to robust Kalman filter. Two kinds of robust Kalman filter are described in detail. The first kind are the one-step methods, which are used directly in real time. The scaled-contaminated model and the methods which uses score functions based on the Bayes' theorem belong to this kind of robust Kalman filter. The second kind are the methods based on the weight or variance iteration of observations, which flow from the adjustment into the Kalman filter and are used in post processing. As examples, two algorithms are constructed with the Huber-function and the Hampel-function respectively. Besides, the robust method based on the a-posteriori estimation of variance components of observations, which was developed by Li [1983], is realized in the Kalman filter in this Chapter.
In Chapter 8, a strategy is presented for a comprehensive post processing of data from the kinematic surveying of land vehicle trajectory.
Chapter 9 summarizes the thesis. |
Numéro de notice : |
61553 |
Affiliation des auteurs : |
non IGN |
Thématique : |
POSITIONNEMENT |
Nature : |
Monographie |
Permalink : |
https://documentation.ensg.eu/index.php?lvl=notice_display&id=60949 |
| 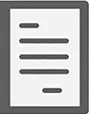 |